Novel AI Paradigm - Equilibrium Machines
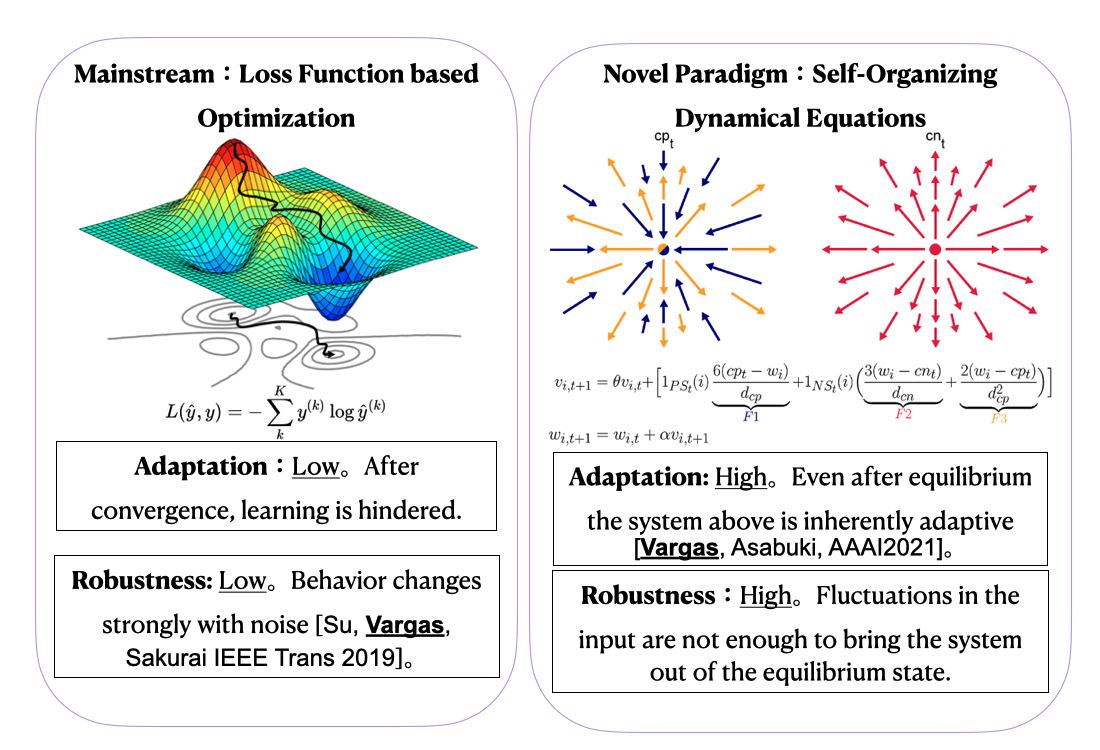
"Would systems be able to learn without optimization?".
Nature seems to rely more on self-organization and extremely non-linear dynamical systems rather than precise optimization, yet natural systems are usually adaptive and robust.
So this question sticked in my mind for a while.
What if it is possible and what would be the foundation of such a learning system?
In 2021, the first answer to the question came with
SyncMap, the first method able to learn based only on dynamical equations that self-organize, no loss function is used.
In fact, its dynamics can be shown to produce multiple loss functions and therefore generalize the concept.
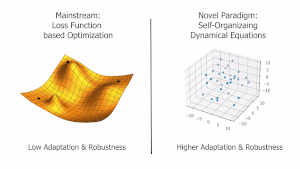
It is inherently adaptive and robust to variations in the input.
This is due to the fact that instead of converging to a maximum or minimum, its learning is based on finding the equilibrium. Equilibrium, however, is by definition a stable property of the system in the presence of noise, which makes it robust to perturbations.
Moreover, by defining a dynamical equation combining both internal dynamics and the external input makes the system intrinsically adaptive. This happens because the equilibrium itself is only defined for the current input structure and structural changes in the input cause, naturally, changes in the attractors of the system which makes it adapt.
We already have results for high-dimensional, hierarchies and many others as the paradigm continues to evolve beyond, surpassing most of my expectations.
Bio-Experiments - Foundations of Intelligence and the Cell
Today, artificial neural networks are perhaps the most widely used AI methods.
It is mostly studied in engineering and computer science fields, however, the first neuron that bootstrapped the area was itself invented by a psychologist.
One can not deny the influence of both neuroscience, psychology and other cognitive science areas have in artificial intelligence.
Here, we dive deeper into biology, neuroscience and epigenetics seeking an understanding of the intelligence.
From an interdisciplinary point of view, we use all tools available including last generation microscopes, stem cells, AI based modeling, mechanical devices among other things to explore and understand the foundations of intelligence.
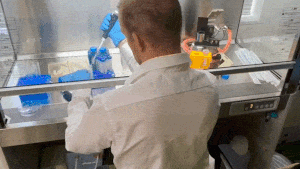
In fact, much is talked about neurons and the brain, but I propose that intelligence is not a property of the neurons or the brain, but of the cell.
The cell is a marvelous entity.
We were once just a cell and even in that stage we could eat, adapt and survive.
Electronic devices need all the pieces to have some function, cellular systems always function.
The cell can repair itself, it can communicate with others, it can reproduce, it is an extremely complex machinery that makes all our human engineering look like primitive developments.
That is why, in this lab, we also seek to understand the cell.
If we can make our devices or algorithms more like the cell, self-sustainability will not be a goal, it will be a foundation.
Deep Neural Network Applications
Given a huge amount of data, deep neural networks can extract complex correlations and predict the most complex phenomena.
In this lab, we have many applications of deep neural networks including persona-based large language models, autonomous driving, image/video generation, etc.
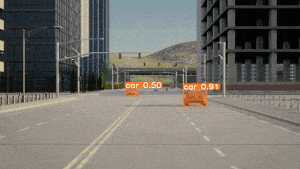
The number of applications is only limited to one's imagination and the presence of large amounts of data.
Current challenges are in dealing with few data (generalization), noisy input (robustness) and varied tasks (adaptation).
Dealing with few data and noisy data would enable the application of deep neural networks to even more real world problems.
Having said that, these are known to be deep neural networks' problems for quite some time.
The research theme "Robust and Adaptive AI" talks about these problems in depth.
Robust and Adaptive AI
Albeit the huge improvement of deep learning methods over the years, there are no robots walking around us.
Deep learning can beat the top professionals in the Go game but lose to amateurs.
Deep learning can surpass humans' accuracy in recognizing images but misclassify when one-pixel is modified.
Indeed, in a research that
appeared on BBC news, we showed that
only one-pixel is necessary to change between classes.
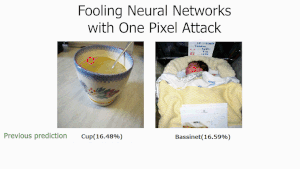
This reveals that deep learning solutions are surprisingly shallow.
While they can learn complex correlations, they also learn unrobust features, trading robustness for accuracy.
For many years we and other researchers have been looking for solutions to these problems.
We found several mechanisms that can explain the problems.
Improvements are existent but are more like patches than real solutions.
The only methods capable of getting near perfect adaptation and robust solutions are based on the "Novel AI Paradigm - Equilibrium Machines" rather than optimization.
Having said that, novel architectures or hybrid systems are still not extensively researched. They could change the current results and pave the way for a new generation of AI.
Novelty-Organizing & Self-Organizing Classifiers
Novelty-Organizing Team of Classifiers (NOTC) is the first method to join both direct policy search and value function approaches into one method.
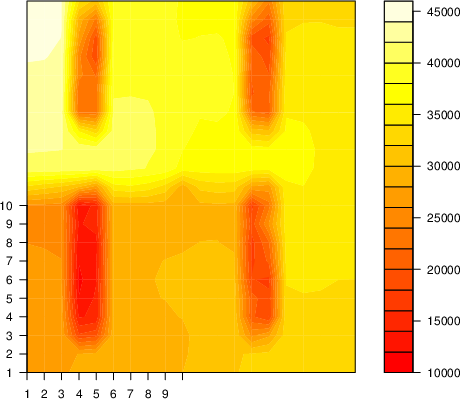
In fact, it is part of a new class of algorithms called
Self-Organization Classifiers which is the first and perhaps the only type of algorithm that can adapt on the fly when a given problem changes (e.g., maze changes in shape).
NOTC's high adaptability is possible because states are automatically updated with experience.

NOTC has also being applied to camera-based autonomous driving and other applications.
It is expected that variations of this algorithm or principles discovered here will be useful in future technologies and/or applications.
SUNA and Unified Neural Model
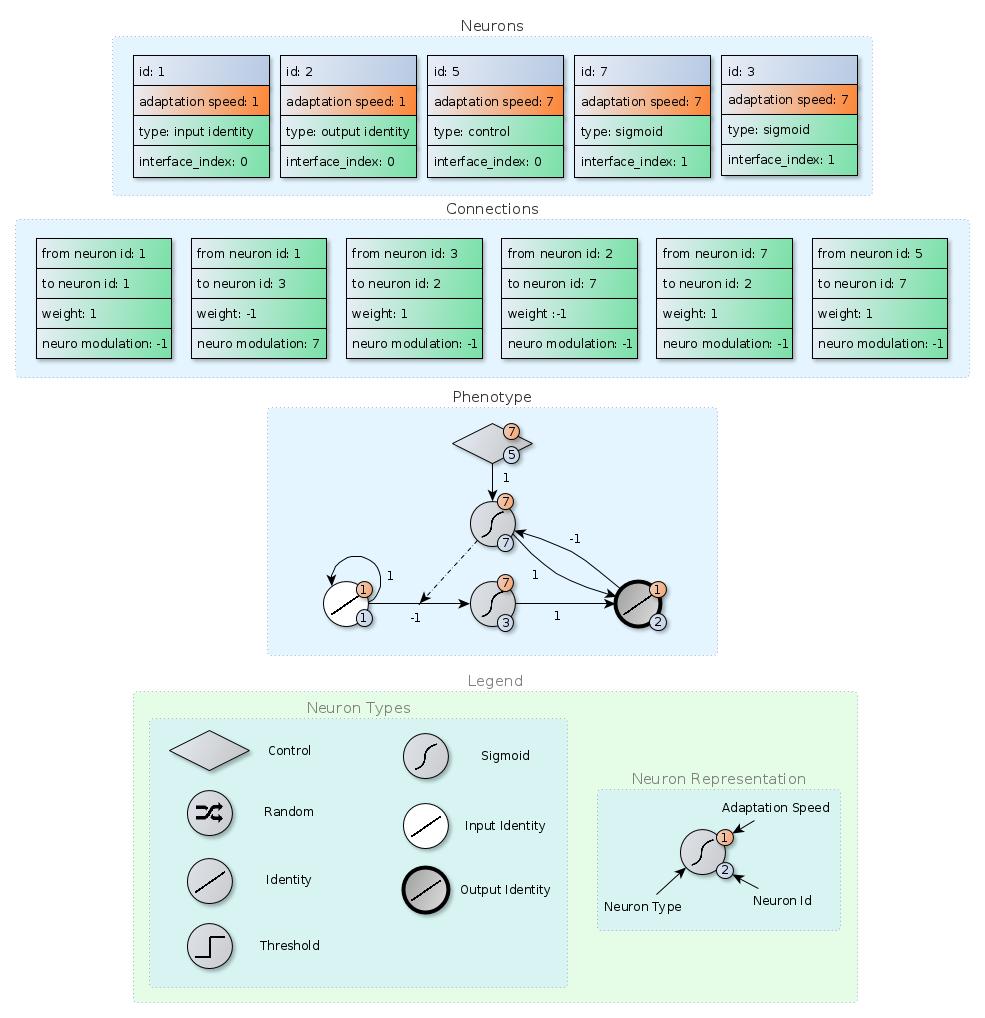
Evolutionary reinforcement learning uses the design flexibility of evolutionary algorithms to tackle the most general paradigm of machine learning, reinforcement learning.
SUNA is the first and currently the only algorithm that takes this flexibility to the limit, unifying most of the previous proposed features into one unified neuron model.
SUNA is a learning system that evolves its own topology and is able to learn even in non-markov problems.
In other words, SUNA's generality increases robustness to parameters and types of problems that can be solved.
SUNA and its variations were applied to various problems including function approximation, neural based symmetric encryption.
Moreover, a method based on SUNA was also applied to the evolution of Deep Neural Networks.
Hacking AI Systems
Hacking into AI systems is one of the few ways to enter their world.
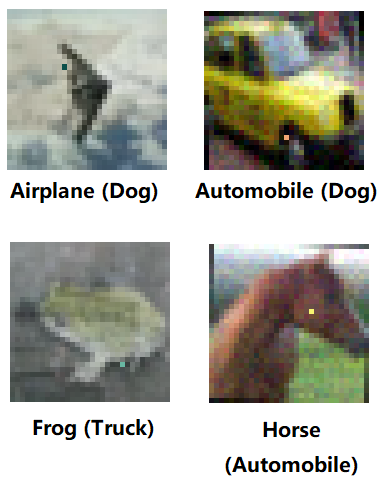
Attacking learning systems allow us to dive deep into the code learned by a system, revealing what a learning system understands to be, for example, a "horse" or a "ship".
Indeed, in a research that
appeared on BBC news, we showed that
only one-pixel is necessary to change between classes.
In other words, the learned concepts of, for example, "horse" or "ship" can be changed with only one pixel.
This demonstrates that although such neural networks achieve suprahuman recognition in datasets what the neural networks really "understand" to be a "horse" or "ship" is far from intelligent.
We will continue to hack AI systems to test and understand them.
For there are a lot of unexplored land inside the black-box of AI systems.
Novel Optimization
SAN is an extremely simple multi-objective optimization algorithm which outperformed all algorithms in the hardest multi-objective benchmark.
When analyzed under the
concept of Optimization Forces it was revealed that the reason for SAN's performance derive from avoiding the conflict inside single population of candidate solutions by using independent subpopulations.
Learning algorithms are nothing but optimization algorithms which allow machines to learn and adapt.
The next generation of machine learning will certainly have a stronger synergy between them and models to be evolved.